教本を参考にKerasで手書き数字画像認識の学習をさせてみました。私の条件では7回目の学習で正解率が99.1%に達しサチりました。
調べてみるとAIフレームワークのTOP3はTensorflow(Google)、PyTorch(Facebook)、Keras(Google系)らしく、それらの中でもTensorflowとPyTorchが抜けた存在だとか。日本産のChainerは開発元がPyTorchを採用・移行し、開発中断となっています。
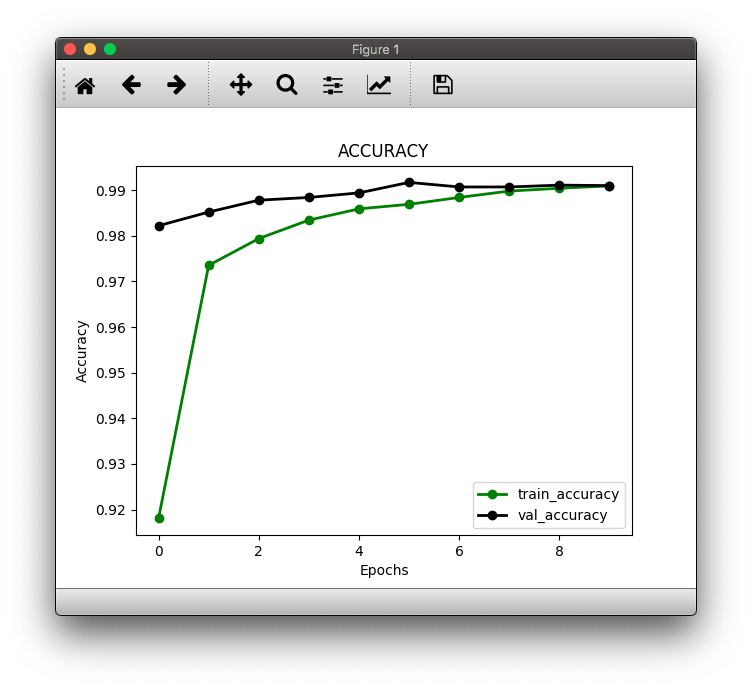
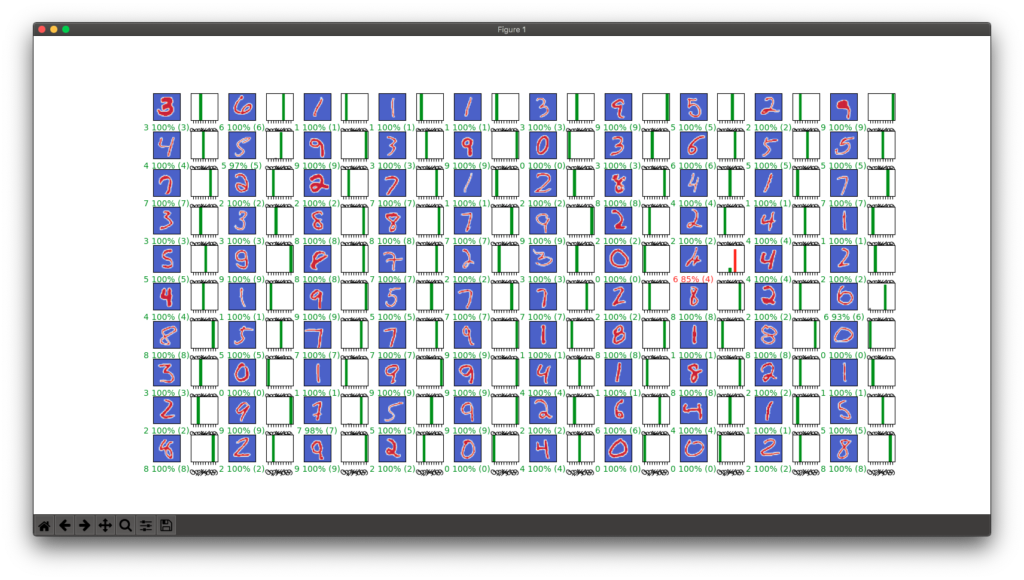
import matplotlib.pyplot as plt
import numpy as np
import keras
from keras.datasets import mnist
from keras import backend as Keras
from keras.models import load_model
NUM_CLASSES = 10
IMG_ROWS, IMG_COLS = 28, 28
def plot_image(data_location, predictions_array, real_teacher_labels, dataset):
predictions_array, real_teacher_labels, img = predictions_array[data_location], real_teacher_labels[data_location], dataset[data_location]
plt.grid(False)
plt.xticks([])
plt.yticks([])
plt.imshow(img,cmap="coolwarm")
predicted_label = np.argmax(predictions_array)
# 文字の色:正解は緑、不正解は赤
if predicted_label == real_teacher_labels:
color = 'green'
else:
color = 'red'
plt.xlabel("{} {:2.0f}% ({})".format(handwritten_number_names[predicted_label],
100*np.max(predictions_array),
handwritten_number_names[real_teacher_labels]),
color=color)
def plot_teacher_labels_graph(data_location, predictions_array, real_teacher_labels):
predictions_array, real_teacher_labels = predictions_array[data_location], real_teacher_labels[data_location]
plt.grid(False)
plt.xticks([])
plt.yticks([])
thisplot = plt.bar(range(10), predictions_array, color="#666666")
plt.ylim([0, 1])
predicted_label = np.argmax(predictions_array)
thisplot[predicted_label].set_color('red')
thisplot[real_teacher_labels].set_color('green')
def convertOneHotVector2Integers(one_hot_vector):
return [np.where(r==1)[0][0] for r in one_hot_vector]
## データの前処理
handwritten_number_names= [str(num) for num in range(0,10)]
# handwritten_number_names = ['0', '1', '2', '3', '4', '5', '6', '7', '8', '9'] # 教本では分かりやすく記述している
(train_data, train_teacher_labels), (test_data, test_teacher_labels) = mnist.load_data()
if Keras.image_data_format() == 'channels_first':
train_data = train_data.reshape(train_data.shape[0], 1, IMG_ROWS, IMG_COLS)
test_data = test_data.reshape(test_data.shape[0], 1, IMG_ROWS, IMG_COLS)
input_shape = (1, IMG_ROWS, IMG_COLS)
else:
train_data = train_data.reshape(train_data.shape[0], IMG_ROWS, IMG_COLS, 1)
test_data = test_data.reshape(test_data.shape[0], IMG_ROWS, IMG_COLS, 1)
input_shape = (IMG_ROWS, IMG_COLS, 1)
train_data = train_data.astype('float32')
test_data = test_data.astype('float32')
print(test_data)
train_data /= 255 # 今回は検証なのでtrain_dataは使いません
test_data /= 255
test_teacher_labels = keras.utils.to_categorical(test_teacher_labels, NUM_CLASSES)
## データの前処理 終了
# 学習モデルの読み込み
model = load_model('keras-mnist-model.h5')
# 予測実行
prediction_array = model.predict(test_data)
# 描画用検証データに変換
test_data = test_data.reshape(test_data.shape[0], IMG_ROWS, IMG_COLS)
# 100個のデータを予測(201-300)
NUM_ROWS = 10
NUM_COLS = 10
NUM_IMAGES = NUM_ROWS * NUM_COLS
START_LOC = 200
plt.figure(figsize=(2*NUM_COLS-4, 1*NUM_ROWS-2))
plt.subplots_adjust(wspace=0.4, hspace=0.4)
for i,num in enumerate(range(START_LOC,START_LOC + NUM_IMAGES)):
plt.subplot(NUM_ROWS, 2*NUM_COLS, 2*i+1)
plot_image(num, prediction_array,convertOneHotVector2Integers(test_teacher_labels), test_data)
plt.subplot(NUM_ROWS, 2*NUM_COLS, 2*i+2)
plot_teacher_labels_graph(num, prediction_array, convertOneHotVector2Integers(test_teacher_labels))
_ = plt.xticks(range(10), handwritten_number_names, rotation=45)
plt.show()