[macOS catalina 10.15.7, Python 3.9.7]
学習モデル作成時の精度等データをJSONファイルに保存できるようにしました。
これで学習モデル間の比較が容易になります。前回記事でソースコードのパスや学習時間をグラフ画像に不可視的に埋め込みましたが、同時にこのファイルの要素として入れておくといいでしょう。
学習モデル作成部分はネットから拝借しました。過学習傾向ですが、精度は高めです。
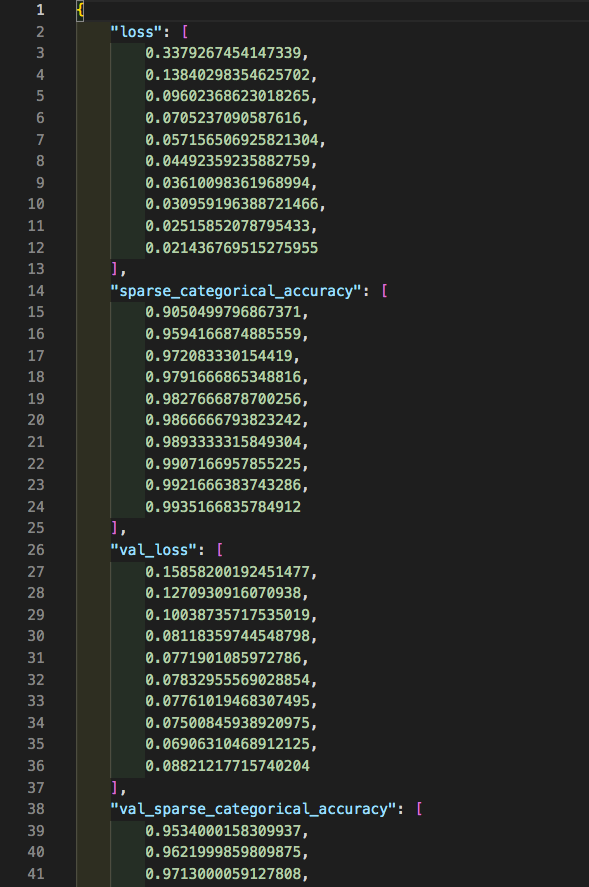
import tensorflow as tf
import json
def plot_loss_accuracy_graph(history):
<略>
return dt_now_str # グラフ作成日時の文字列
def create_model():
model = models.Sequential()
model.add(layers.Input((784,)))
model.add(layers.Dense(128, activation="relu"))
model.add(layers.Dense(64, activation="relu"))
model.add(layers.Dense(10, activation="softmax"))
return model
def main():
# 前処理
(x_train, y_train), (x_test, y_test) = tf.keras.datasets.mnist.load_data()
x_train = x_train.astype(np.float32).reshape(-1, 784) / 255.0
x_test = x_test.astype(np.float32).reshape(-1, 784) / 255.0
# 学習モデル作成
model = create_model()
loss = tf.keras.losses.SparseCategoricalCrossentropy()
acc = tf.keras.metrics.SparseCategoricalAccuracy()
optim = tf.keras.optimizers.Adam()
model.compile(optimizer=optim, loss=loss, metrics=[acc])
# 学習
epochs = 10
history = model.fit(x_train, y_train, validation_data=(x_test, y_test), epochs=epochs, batch_size=128)
print(f'history.history {history.history}')
# 学習データグラフ化
ret = plot_loss_accuracy_graph(history)
print(f'history.history {history.history}')
# データ保存
json_file = '{}_history_data.json'.format(ret)
with open(json_file ,'w' ) as f:
json.dump(history.history ,f ,ensure_ascii=False ,indent=4)
# 検証結果
test_loss = history.history['val_loss'][-1]
test_accuracy = history.history['val_sparse_categorical_accuracy'][-1]
print('Test loss:', test_loss)
print('Test accuracy:', test_accuracy)
model.save('keras-mnist-model.h5')
if __name__ == "__main__":
start = time.time()
dt_now = datetime.datetime.now()
print('処理開始 '+ str(dt_now))
main()
# 処理時間算出
process_time = time.time() - start
td = datetime.timedelta(seconds = process_time)
dt_now = datetime.datetime.now()
print('処理終了 ' + str(td) + ' ' + str(dt_now))